As policymakers across the globe approach regulating artificial intelligence (AI), there is an emerging and welcomed discussion around the importance of securing AI systems themselves. Indeed, many of the same governments that are actively developing broad, risk-based, AI regulatory frameworks have concurrently established AI safety institutes to conduct research and facilitate a technical approach to increasing AI system resilience.
Much of the early work of these AI safety institutes has understandably focused on the cybersecurity of the most powerful large language models (LLMs) and generative AI systems, collectively referred to here as GenAI. These models are increasingly being integrated into applications and networks across every sector of the economy. This is why it’s important for policymakers to understand the unique risks facing the GenAI ecosystem and the mitigation strategies needed to bolster GenAI security as they are adopted.
Over the past few years, Palo Alto Networks has been on the front lines, working to understand these threats and developing security approaches and capabilities to mitigate them. A key pillar of this work has been the development of a GenAI cybersecurity framework, comprising five core security aspects. Each outlines the challenges and attack vectors across the different stages of GenAI security. (See figure below.)
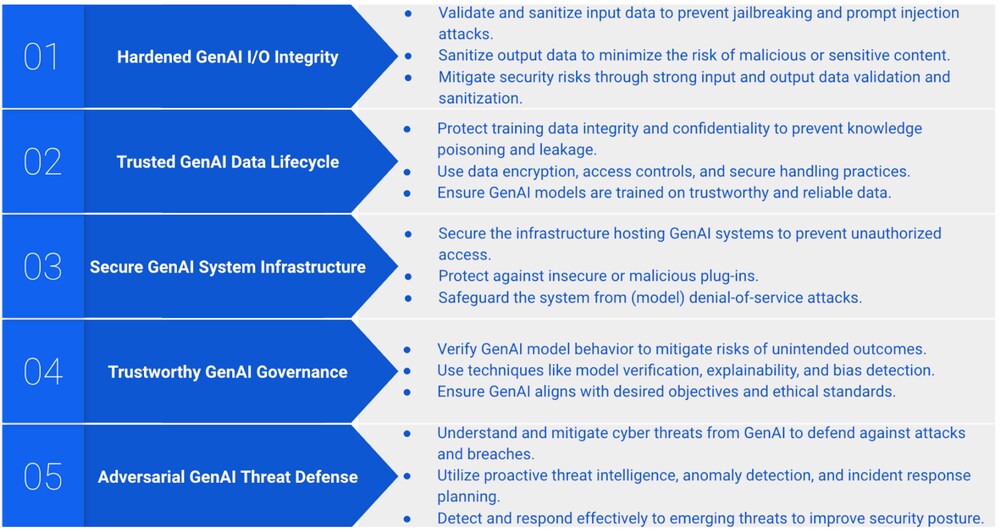
Central to our GenAI cybersecurity framework is the need to address the full lifecycle of secure and responsible GenAI development and use. This entails understanding the threats to these systems, developing tactics to detect incidents and compromises, and implementing capabilities to secure the AI lifecycle by design.
Threats to AI Systems
It’s important for enterprises to have visibility into their full AI supply chain (encompassing the software, hardware and data that underpin AI models) as each of these components introduce potential risks. A supply chain attack, targeting a third-party code library, could potentially impact a wide range of downstream entities. To mitigate these risks, companies should consider adopting a Zero Trust network architecture that enables continuous validation of all elements within the AI system. Regularly updating dependency mapping, monitoring the integrity of AI models, and securing cloud environments where AI systems are hosted are also key strategies in securing the AI supply chain.
Adversarial attacks on GenAI systems can also manipulate input data in a way that results in AI models subsequently making incorrect predictions or classifications. For example, a slightly modified image file could cause an AI model to misidentify an object, with potentially serious impacts in use cases, like autonomous driving. To protect against these unintended outcomes, robust defenses, like adversarial training where models are trained using both clean and adversarial threat signatures, can be deployed to help improve resilience. Data encryption, secure transmission protocols and continuous monitoring for unusual patterns in AI system behavior are also recommended safeguards.
Incident Detection and Response
The importance of establishing a robust threat detection and incident response strategy for AI systems cannot be overstated. AI systems need to be designed with recoverability in mind, ensuring that compromised models can be quickly isolated and replaced with trusted backups to minimize disruption.
Since AI systems are often more dynamic than legacy IT environments, making them susceptible to unique threats, it’s important to monitor model behavior for signs of compromise or tampering. This monitoring can be assisted with robust AI system logging, which helps track and analyze anomalies that may indicate security breaches.
Secure AI by Design
The concept of securing AI systems by design is Foundational to AI security. This approach shifts the focus from retroactive security measures to proactive and intentional architecture that incorporates security into every stage of AI development and deployment. To that end, any framework for securing AI systems should encourage organizations to:
- Discover, Classify and Govern AI Applications – Implementing processes and/or adopting tools to identify all AI-powered applications that are running within an organization's infrastructure gives security professionals different abilities.
- Gain visibility and control over hundreds of known and unknown third-party AI applications running in their environment.
- Help prevent sensitive data leaks with comprehensive data classification capabilities.
- Continue to secure their devices, applications and networks against threats originating from insecure or compromised AI platforms.
- Protect AI Applications in Runtime – Continuously monitoring and securing AI applications that are being used within an organization’s environment is known as “runtime” security. This security posture enables information security professionals to protect those AI applications from unique AI threats, like prompt injection attacks, data poisoning attacks and LLM denial-of-service attacks.
- Secure the AI Development Supply Chain – Addressing security risks in the AI development supply chain can help AI developers address unique AI-based threats and vulnerabilities. Frameworks should advance processes and adopt tools that help those developers gain better visibility into the AI application code, and to identify the lineage of AI components and data used in building applications. Such a posture will enable developers to reduce data exposure and identify misconfigurations.
AI Security Complements Ethical Model Use Imperatives
As AI systems often process large amounts of personal and sensitive data, ensuring privacy becomes a significant concern. Fortunately, there are techniques, such as differential privacy, that allows AI systems to learn from data without revealing personal information, that can advance both privacy protection and data security goals. In a similar vein, by applying noise to datasets, companies can ensure that individual user data remains anonymous while still allowing for meaningful insights to be extracted by the GenAI model.
Looking Forward
As AI systems, both GenAI and more traditional machine learning or inline learning models, continue to evolve, so too will the threats they face. Recognizing this backdrop, any regulatory or policy framework for AI must ensure that security remains a continuous priority throughout the lifecycle of AI systems. This will help foster better collaboration between government officials, AI developers and the cybersecurity communities to stay ahead of emerging threats.
Acknowledgments: Thanks to the outstanding researchers, engineers and technical drafting team that developed the original six-blog series on the Palo Alto Networks GenAI Security Framework, including Royce Lu, Bo Qu, Yu Fu, Yiheng An, Haozhe Zhang, Qi Deng, Brody Kutt, Nicole Nichols, Katie Strand and Aryn Pedowitz. That series is available on Palo Alto Networks LIVEcommunity blog page.